Thinking
The role of UX: point AI at problems worth solving
In previous articles, we've shared some of the takeaways from our conversations with Josh Clark and Veronika Kindred about the future of design and AI. But the most important point that guides our approach to integrating AI into projects is this: use AI to solve problems that matter.
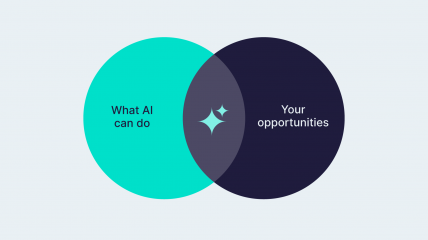
In a recent paper on customer experience in the age of AI, KPMG outlined several risks in implementing AI in innovation projects. These stood out in particular:
- Narrow definitions of value focused on cost, missing customer benefits
- Multiple AI models and "use cases" lead to disconnected experiences
- IT-first AI strategies, without mature business or customer leadership
Our human-centred approach to innovation drives us to understand the context before introducing technological change, and the more we reflect on these points, the more confident we are in our direction.
Especially with emerging technologies and uncertain market conditions, planning investments carefully and managing risk is critical. The first step is to clarify what stage of innovation we're in.
Understand where you are: read the context before designing
It's not just about starting with the problem, but about having a clear view of the system we’re operating in. As I wrote in a previous post in this series, "Build the right product before building the product right". Or, to quote a very insightful article I recently read: in an era where the cost of answers tends toward zero, the quality of the questions becomes infinitely more important.
First of all, what phase of innovation are we in?
Are we adding intelligence to an existing product or service? Are we creating something new with AI elements? Or are we trying to build an AI-native product?
Are we pursuing a strategy of efficiency and automation, aiming to improve customer experience, or stepping into reinvention? These questions help us set the right expectations.
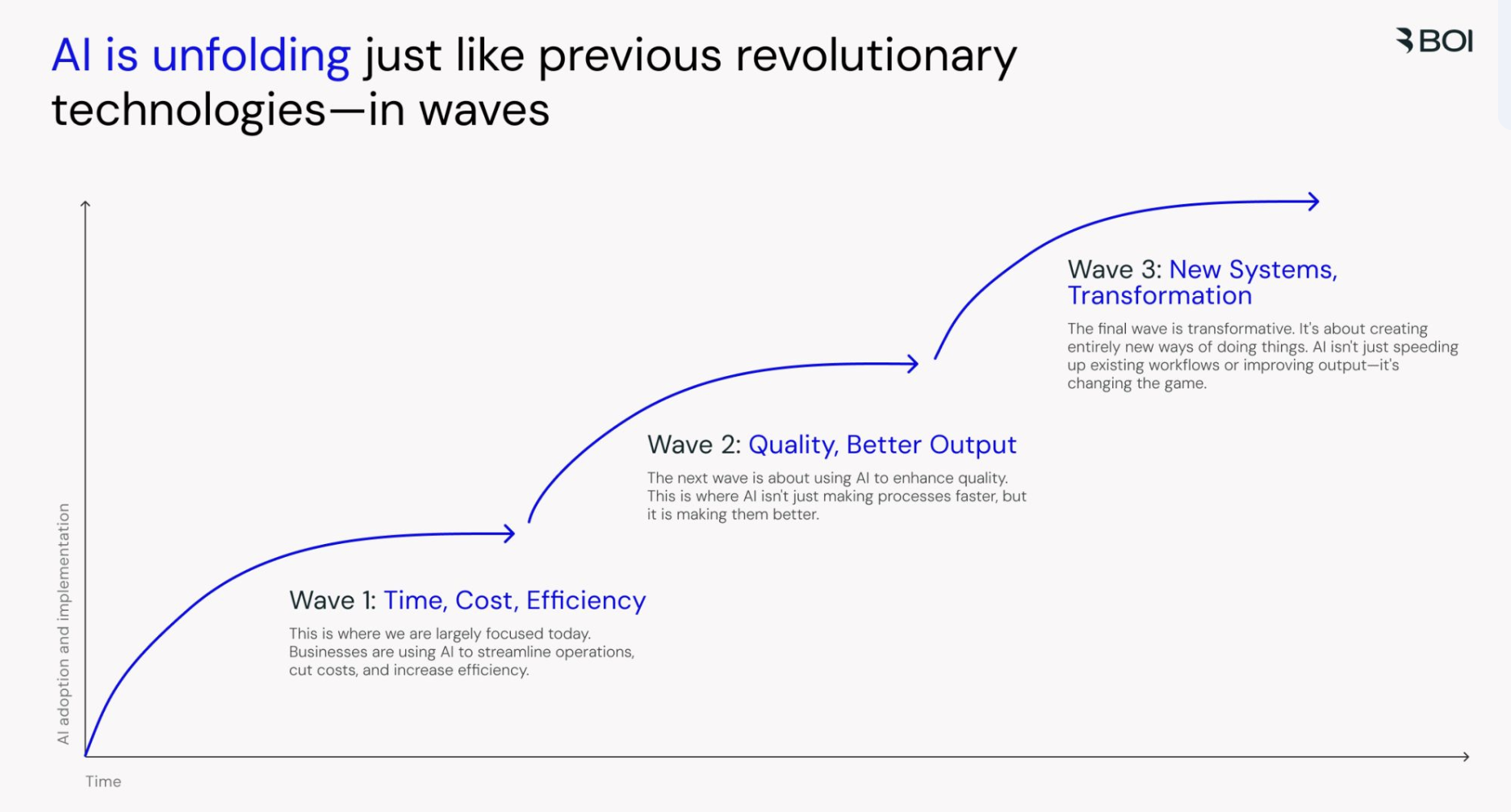
Naturally, we also need to map who our users are (or better yet, all system actors, not just users), what the use contexts are, the tasks and actions, and any other information to give us a clear picture of the scenario we're working in.
Problem-framing has always been important, but in this case, it's especially so: it helps avoid aiming AI at low-value problems or expecting magical results without the necessary foundations in place. Once the scope is defined, we can start looking for meaningful opportunities to apply AI.
Where can AI make a difference?
Especially in the most common scenario — integrating AI into existing products and services — the next step is to identify opportunities. Josh Clark’s conceptual framework is a useful guide here:
- What results are we aiming for?
- What causes friction or gets in the way today?
- What assumptions are we working with?
- How can AI help?
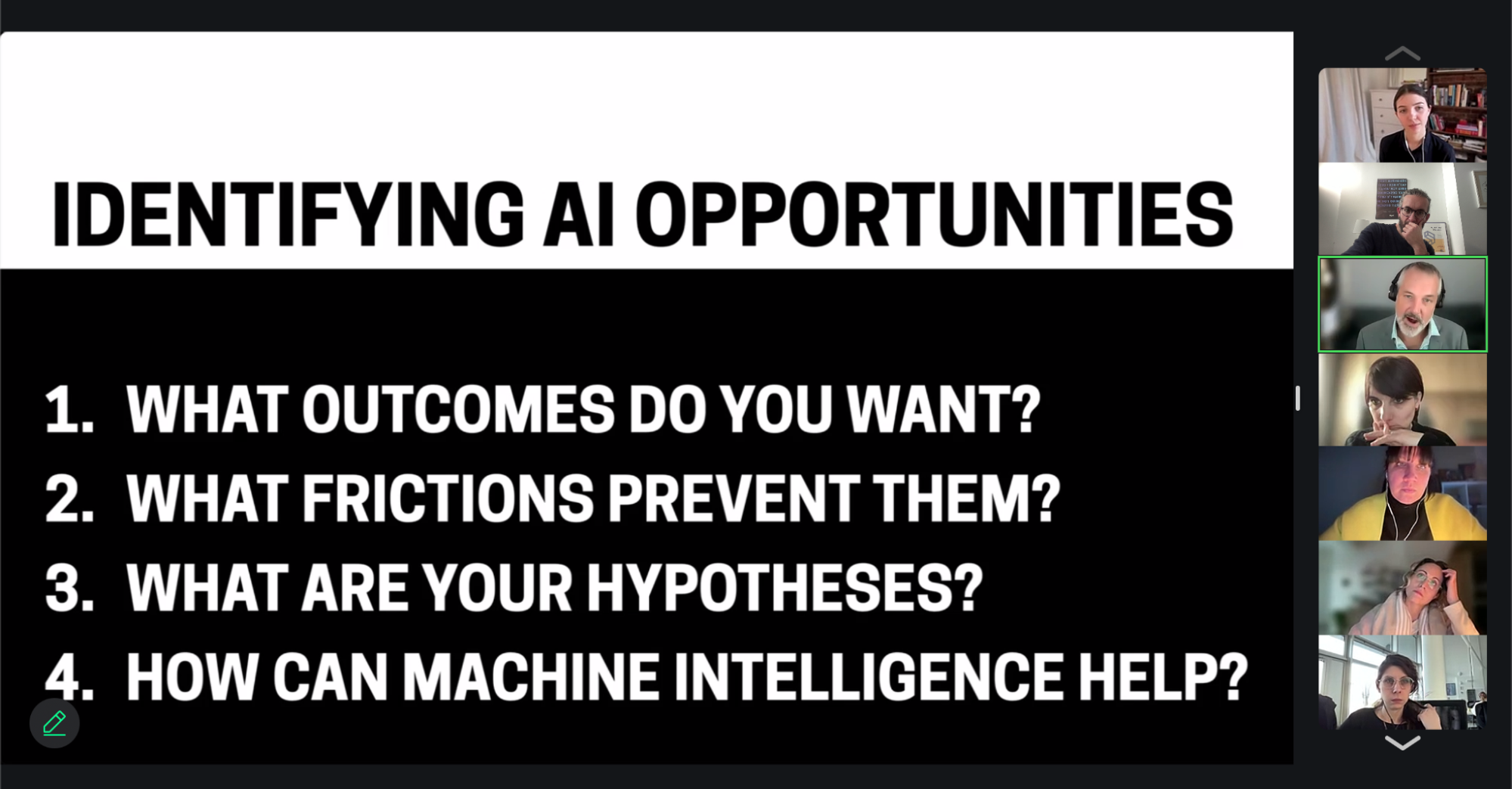
Another way to generate ideas is to work from the customer journey of the current experience. By mapping it, we can identify points where automation, intelligence or personalisation could improve the experience. This applies to both pain points and areas where we can build on existing strengths or competitive advantages. It's not just about fixing problems - we can also imagine more ambitious, forward-looking scenarios.
At this stage, we need a good dose of divergent thinking and co-design to break out of habitual patterns and standard interaction models. This is where we can plant the seeds of something new while remaining anchored in the context we've analysed.
During this ideation phase, it's also helpful to capture any thoughts about trust and transparency. How can we make it easy and attractive for people to adopt this solution? Where there is friction, should we reduce it, or might it serve a purpose, such as encouraging more mindful engagement? How much autonomy do we want to give the system?
There's often a fine line between making an experience seamless and making it meaningful. Efficiency isn't always the answer.
How do we decide what ideas to prioritize?
Through prioritisation and impact assessment, we can narrow down the ideas and decide where to focus.
Depending on the context and strategy, we may opt for more radical ideas, even if they require significant effort, or we may opt for quick wins that are easier and faster to implement. For many existing products and services, it can be useful to look for low to medium-effort ideas that are genuinely helpful and feasible. This is where Quiet Intelligence or Casual Intelligence scenarios often emerge.
At this point, we should have enough information to define what we're trying to build and outline some possible scenarios. This also helps us think about data (which is essential) and the enabling technologies we might need.
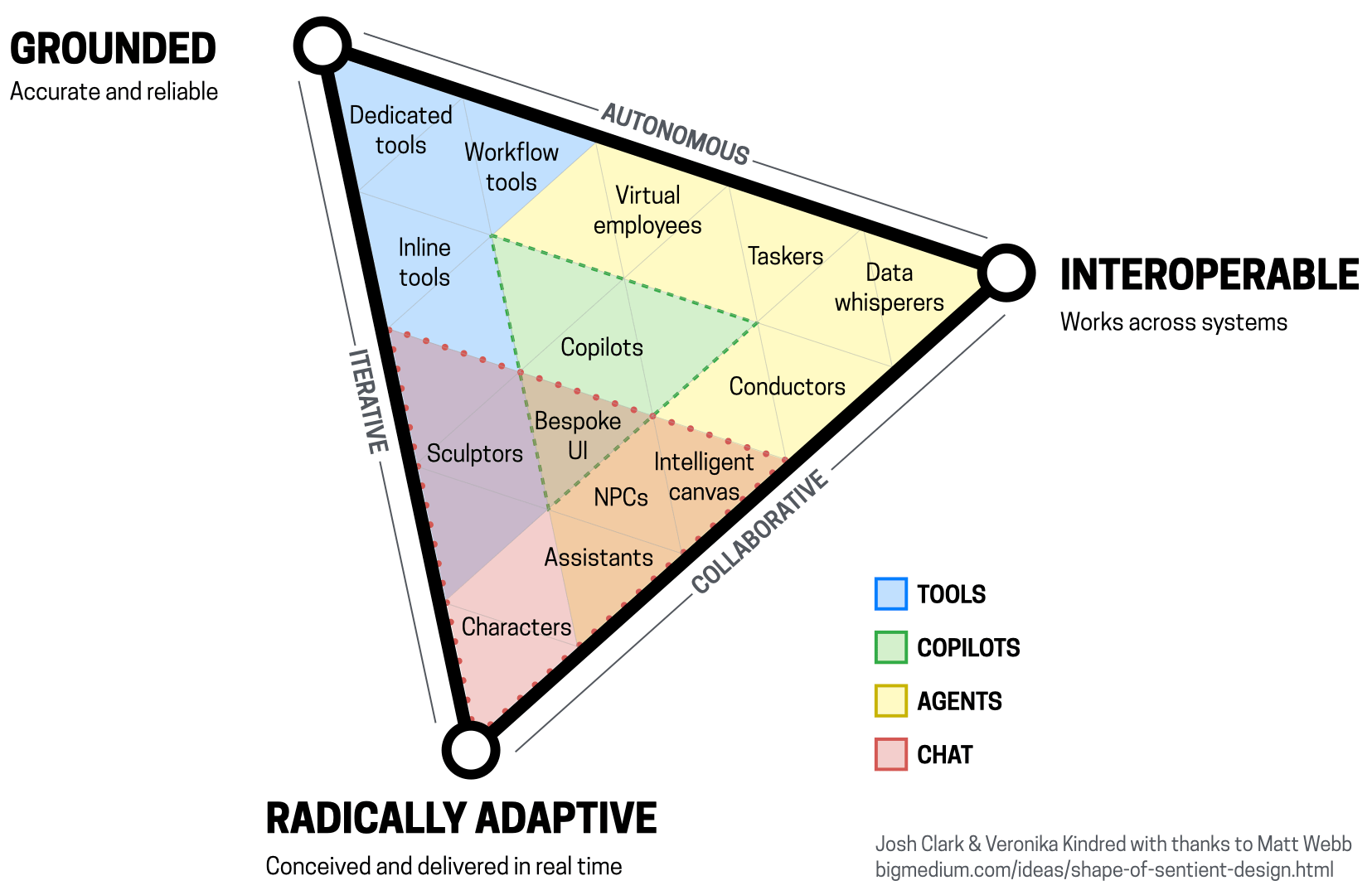
This part of the process is where having a domain expert at the table is absolutely critical.
We typically have these conversations with our partners at LAIF, who help us explore the technology side, from data to model selection.
Test before you build
We are now moving into prototype and proof of concept (POC) territory, but only after going through proper problem framing and idea generation. This process gives us both shared knowledge and a backlog of concepts (and the ideas we leave behind? Great backlog material 😉).
What hypotheses have we formed? How can we test them? Where is the greatest uncertainty and what evidence do we need to move forward? What technological hurdles do we want to be sure we can overcome?
These are some of the questions that will help guide us to the right type of prototype or POC that will provide the answers we need before moving forward or scaling the solution.
Adoption and organization: the real test
This is a crucial factor, whether we're designing a user-facing solution or one for internal teams. Without adoption, even the most brilliant solution will ultimately fail. In the former case, we need to consider users' willingness and ability to adopt new features and build trust in the system - balancing simplicity with intentional design, and information density with decision clarity.
In internal use cases, adoption is even more delicate, as it intersects with organisational dynamics and capabilities. Again, a POC or small-scale experiment can be useful, coupled with a clear strategy for a phased roll-out.
This may be a slower phase, but it's essential to increase the project's chances of success.
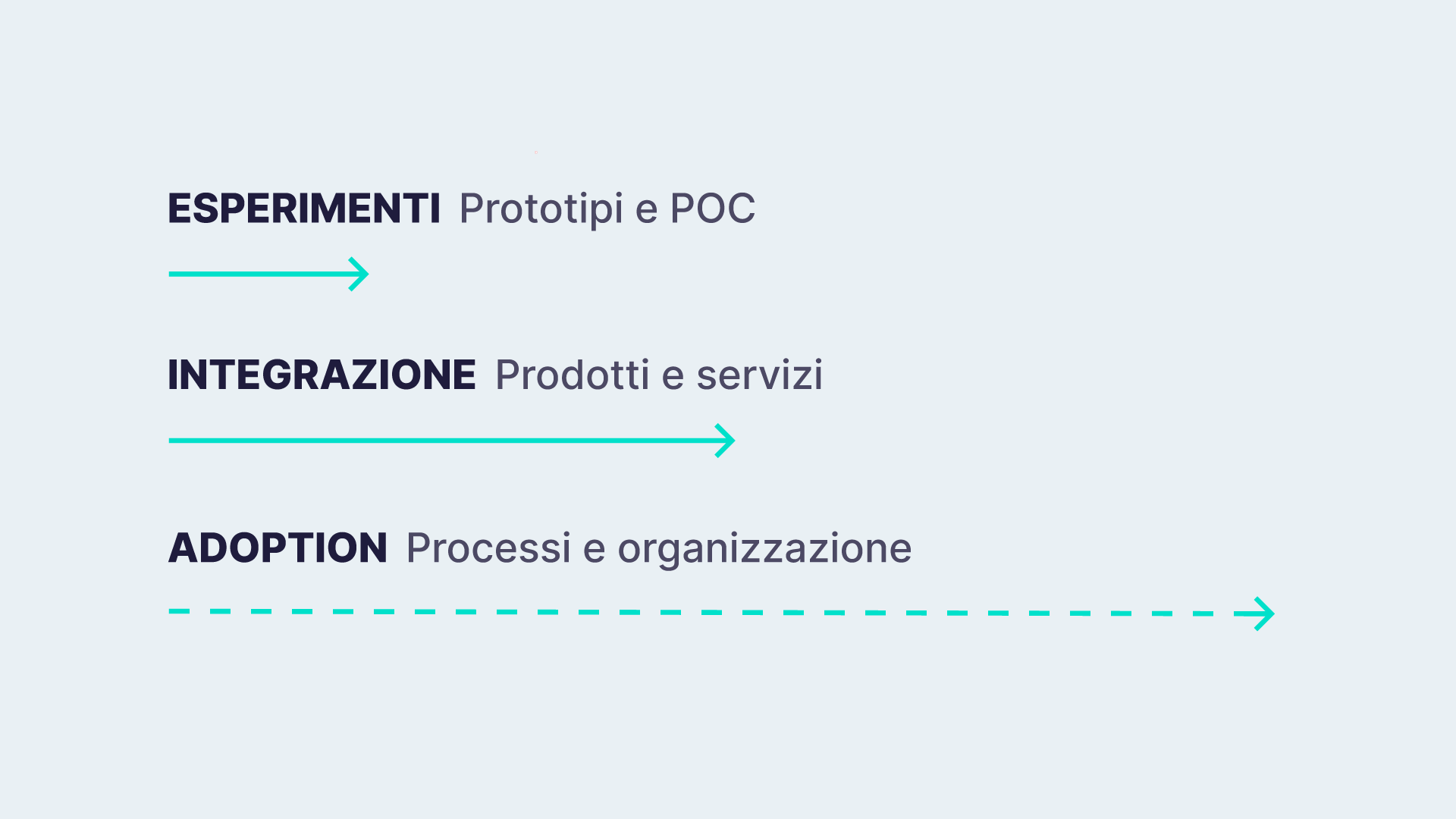
Ready to start? we’ve designed a dedicated workshop
Considering all these factors, we asked ourselves: as designers, how can we help organizations embed AI in a responsible, useful, and sustainable way? At Tangible, we’re developing an approach that starts from contextual understanding, goes through co-design and prioritization, and ends with prototyping and adoption.
This whole process forms the basis of the workshop we've created to help teams navigate between opportunities, technologies and adoption strategies.
If you're exploring the role of AI in your product or service, or designing something new and "smart", let's talk.